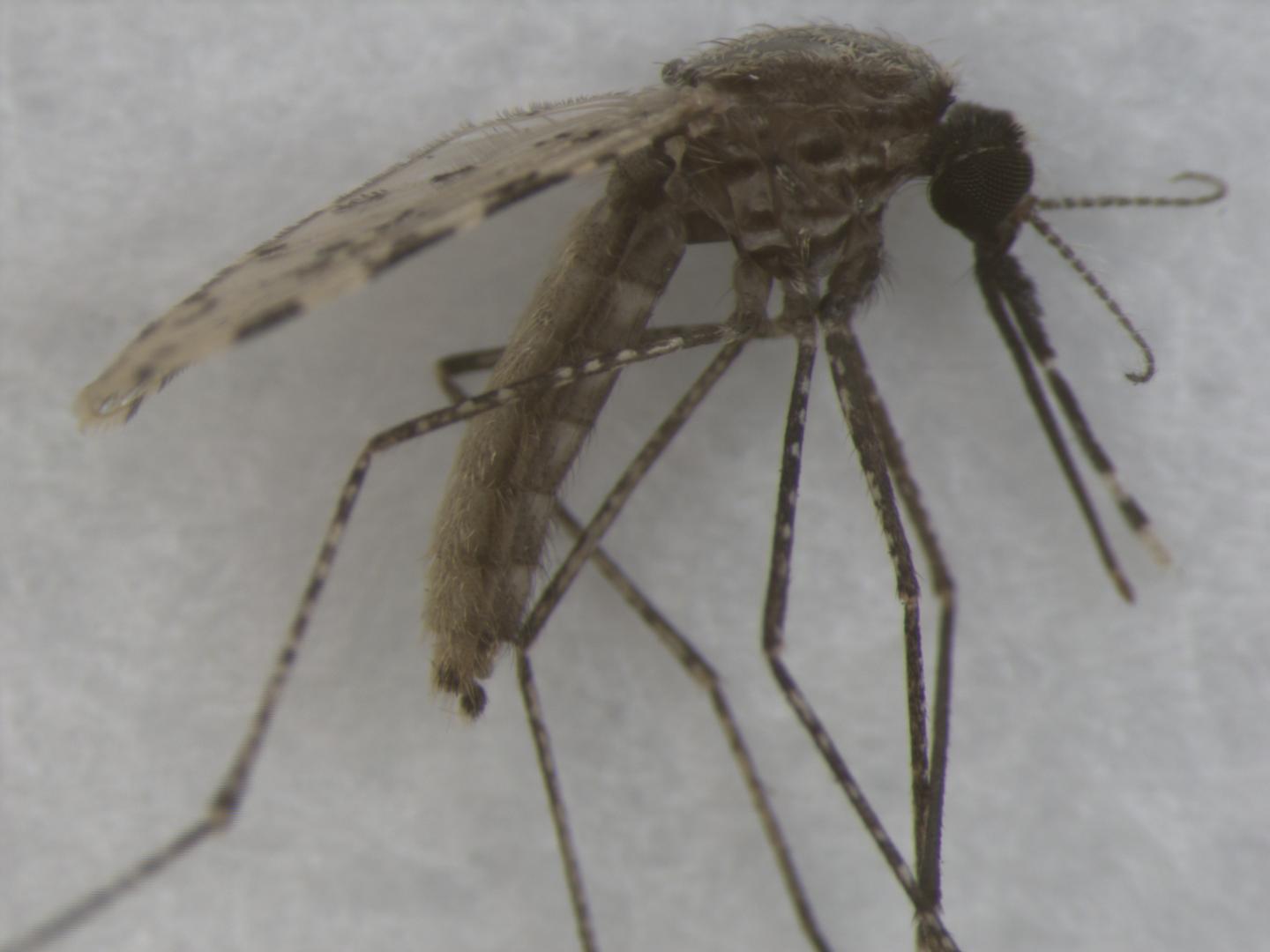
Photo: Representative specimen image of Anopheles stephensi from Mosquito Image Database. Picture shows a mosquito stored by freezing at -80C.
view more
Credit Image: Couret, J. et al. 2020 (CC-BY 2.0)
Rapid and accurate identification of mosquitoes that transmit human pathogens such as malaria is an essential part of mosquito-borne disease surveillance. Now, researchers reporting in PLOS Neglected Tropical Diseases have shown the effectiveness of an artificial intelligence system–known as a Convoluted Neural Network–to classify mosquito sex, genus, species and strain.
Human malaria is an ongoing public health crisis affecting multiple continents, with the highest numbers of cases and people at risk occurring in sub-Saharan Africa. However the identification of mosquitoes that transmit malaria can be difficult–some species are nearly indistinguishable even to trained taxonomists.
In the new work, Jannelle Couret of University of Rhode Island, USA, and colleagues applied a Convoluted Neural Network (CNN) to a library of 1,709 two-dimensional images of adult mosquitos. The mosquitoes were collected from 16 colonies in five geographic regions and included one species not readily identifiable to trained medical entomologists. They also included mosquitoes that had been stored in two different ways–by flash freezing or as dried samples.
Using the library of identified species, the researchers trained the CNN to distinguish Anopheles from other mosquito genera, to identify species and sex within Anopheles, and to identify two strains within a single species. They found a 99.96% prediction accuracy for class and a 98.48% accuracy for sex.
“These results demonstrate that image classification with deep learning can be a useful method for malaria mosquito identification, even among species with cryptic morphological variation,” the researchers say. “The development of an independent and accurate method of species identification can potentially improve mosquito surveillance practices.”
###
Research Article
Peer-reviewed; Simulation / modelling
In your coverage please use this URL to provide access to the freely available paper: http://journals.
Citation: Couret J, Moreira DC, Bernier D, Loberti AM, Dotson EM, Alvarez M (2020) Delimiting cryptic morphological variation among human malaria vector species using convolutional neural networks. PLoS Negl Trop Dis 14(12): e0008904. https:/
Funding: This work was supported by the USDA National Institute of Food and Agriculture, Hatch Regional Project 1021058. The funders had no role in study design, data collection and analysis, decision to publish, or preparation of the manuscript.
Competing Interests: The authors have declared that no competing interests exist.
TDnews (tunisiesoir.com)